Homepage
•
Learning Library
•
Blog
•
Computational Thinking and Data Analysis Go Hand-in-Hand
Expand breadcrumbs
Expand breadcrumbs
- Learning Library
- Blog
- Computational Thinking and Data Analysis Go Hand-in-Hand
- Homepage
- •
- Learning Library
- •
- Blog
- •
- Computational Thinking and Data Analysis Go Hand-in-Hand
Computational Thinking and Data Analysis Go Hand-in-Hand
By Nick Pinder
March 28, 2022
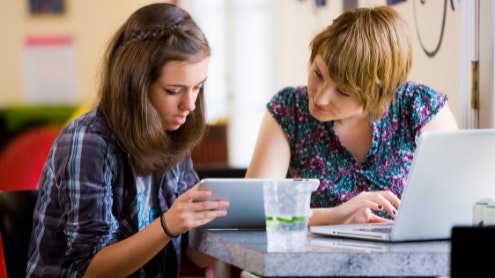